Matrix Decomposition
本文试图用最简洁清晰的语言解释各种矩阵分解,只记录矩阵分解的基础知识和过程,对性质和应用的讨论先挖个坑,下次再填
有关矩阵分解的知识可以参考这个
为什么要矩阵分解?为了更好的计算,降低计算复杂度
Definitions
Unitary matrix
Conjugate transpose
共轭转置 aka Hermitian transpose,计$A^H$为A的共轭转置(有时也写成$A^*$)
对实矩阵,${\displaystyle {\boldsymbol {A}}^{\mathrm {H} }={\boldsymbol {A}}^{\mathsf {T}}}$
对矩阵A,共轭转置后,每个元素的定义为
$${\displaystyle \left({\boldsymbol {A}}^{\mathrm {H} }\right)_{ij}={\overline {{\boldsymbol {A}}_{ji}}}}$$
比如矩阵A
$$ {\displaystyle {\boldsymbol {A}}={\begin{bmatrix}1&-2-i&5\\1+i&i&4-2i\end{bmatrix}}} $$
$$ {\displaystyle {\boldsymbol {A}}^{\mathsf {T}}={\begin{bmatrix}1&1+i\\-2-i&i\\5&4-2i\end{bmatrix}}} $$
$$ {\displaystyle {\boldsymbol {A}}^{\mathrm {H} }={\begin{bmatrix}1&1-i\\-2+i&-i\\5&4+2i\end{bmatrix}}} $$
酉矩阵的定义为,对于方阵U,满足如下性质,U*是共轭转置,I是单位阵
$$ {\displaystyle U^{*}U=UU^{*}=UU^{-1}=I} $$
酉矩阵的行列式绝对值为1,在特征空间中正交,当矩阵为实数阵时,就是正交矩阵(orthogonal matrix)
正交矩阵就是各列向量线性无关,是实数下的酉矩阵
Definite matrix
Definitions for real matrices
假设M是n阶实对称矩阵
$$ {\displaystyle M{\text{ positive-definite}}\quad \iff \quad \mathbf {x} ^{\textsf {T}}M\mathbf {x} >0{\text{ for all }}\mathbf {x} \in \mathbb {R} ^{n}\setminus \{\mathbf {0} \}} $$
$$ {\displaystyle M{\text{ positive semi-definite}}\quad \iff \quad \mathbf {x} ^{\textsf {T}}M\mathbf {x} \geq 0{\text{ for all }}\mathbf {x} \in \mathbb {R} ^{n}} $$
$$ {\displaystyle M{\text{ negative-definite}}\quad \iff \quad \mathbf {x} ^{\textsf {T}}M\mathbf {x} <0{\text{ for all }}\mathbf {x} \in \mathbb {R} ^{n}\setminus \{\mathbf {0} \}} $$
$$ {\displaystyle M{\text{ negative semi-definite}}\quad \iff \quad \mathbf {x} ^{\textsf {T}}M\mathbf {x} \leq 0{\text{ for all }}\mathbf {x} \in \mathbb {R} ^{n}} $$
Definitions for complex matrices
设M是n阶Hermite复矩阵
$$ {\displaystyle M{\text{ positive-definite}}\quad \iff \quad \mathbf {x} ^{*}M\mathbf {x} >0{\text{ for all }}\mathbf {x} \in \mathbb {C} ^{n}\setminus \{\mathbf {0} \}} $$
$$ {\displaystyle M{\text{ positive semi-definite}}\quad \iff \quad \mathbf {x} ^{*}M\mathbf {x} \geq 0{\text{ for all }}\mathbf {x} \in \mathbb {C} ^{n}} $$
$$ {\displaystyle M{\text{ negative-definite}}\quad \iff \quad \mathbf {x} ^{*}M\mathbf {x} <0{\text{ for all }}\mathbf {x} \in \mathbb {C} ^{n}\setminus \{\mathbf {0} \}} $$
$$ {\displaystyle M{\text{ negative semi-definite}}\quad \iff \quad \mathbf {x} ^{*}M\mathbf {x} \leq 0{\text{ for all }}\mathbf {x} \in \mathbb {C} ^{n}} $$
正定矩阵的几何意义参考此处
实际上$x^TAx$的形式表示二次型(有一个概念叫合同与之相关),而根据定义,正定矩阵表示对所有的非零解,它的值都是大于0的,这在空间上是一个开口向上的抛物面(三维),正定矩阵如果看做一种空间变换,就相当于把一块布的四个角往上提了
假设$y=x^TAx$,那么y>0,且y有最小值
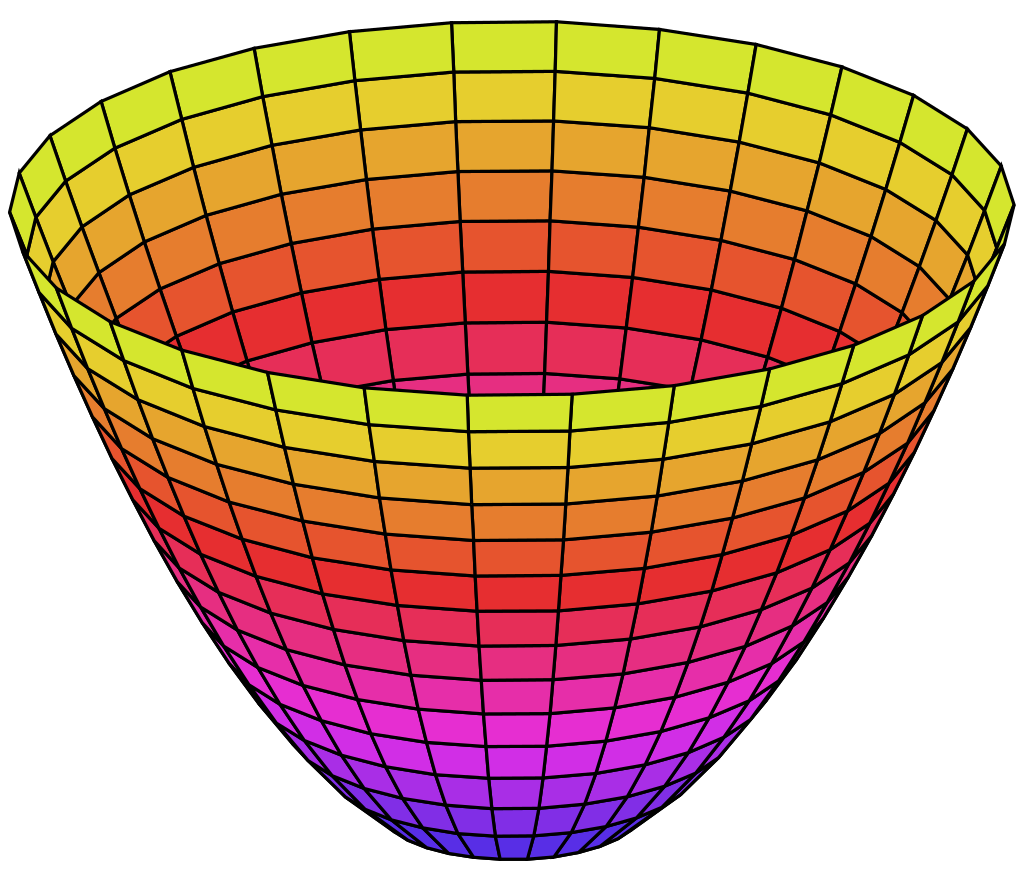
当我们求一个二次型的极值时,假设它是正定矩阵,那么一定有最小值(如上图),而根据泰勒公式,Hessian矩阵就是二次型的矩阵A,所以判断Hessian矩阵是不是正定矩阵就行
Example
单位阵是正定矩阵也是正半定矩阵
$$ {\displaystyle\mathbf{z}^{\textsf{T}}I\mathbf{z}={\begin{bmatrix}a&b\end{bmatrix}}{\begin{bmatrix}1&0\\0&1\end{bmatrix}}{\begin{bmatrix}a\\b\end{bmatrix}}=a^{2}+b^{2}.} $$
这个矩阵M也是正定矩阵
$$ {\displaystyle M={\begin{bmatrix}2&-1&0\\-1&2&-1\\0&-1&2\end{bmatrix}}} $$
$$ {\displaystyle {\begin{aligned}\mathbf {z} ^{\textsf {T}}M\mathbf {z} =\left(\mathbf {z} ^{\textsf {T}}M\right)\mathbf {z} &={\begin{bmatrix}(2a-b)&(-a+2b-c)&(-b+2c)\end{bmatrix}}{\begin{bmatrix}a\\b\\c\end{bmatrix}}\\&=(2a-b)a+(-a+2b-c)b+(-b+2c)c\\&=2a^{2}-ba-ab+2b^{2}-cb-bc+2c^{2}\\&=2a^{2}-2ab+2b^{2}-2bc+2c^{2}\\&=a^{2}+a^{2}-2ab+b^{2}+b^{2}-2bc+c^{2}+c^{2}\\&=a^{2}+(a-b)^{2}+(b-c)^{2}+c^{2}\end{aligned}}} $$
这个矩阵N不是正定矩阵
$$ {\displaystyle N={\begin{bmatrix}1&2\\2&1\end{bmatrix}}} $$
$$ {\displaystyle {\begin{bmatrix}-1&1\end{bmatrix}}N{\begin{bmatrix}-1&1\end{bmatrix}}^{\textsf {T}}=-2<0} $$
Eigenvalues
Type | Condition(iff all eigenvalues are) |
---|---|
positive definite | positive |
positive semi-definite | non-negative |
negative definite | negative |
negative semi-definite | non-positive |
indefinite | both positive and negative |
Hermitian matrix
共轭转置为自身的方阵叫Hermite矩阵
$$ {\displaystyle A{\text{ Hermitian}}\quad \iff \quad a_{ij}={\overline {{a}_{ji}}}} $$
或者
$$ {\displaystyle A{\text{ Hermitian}}\quad \iff \quad A=A^{\mathsf {H}}} $$
Hermite矩阵的对角线必须为实数,如下就是一个Hermite矩阵,由此可见对称矩阵一定是Hermite矩阵
$$ {\displaystyle {\begin{bmatrix}0&a-ib&c-id\\a+ib&1&m-in\\c+id&m+in&2\end{bmatrix}}} $$
Eigenvalues and eigenvectors
此处可以去看3b1b的视频,讲解非常清晰
只有方阵才有特征值和特征向量
方阵的秩不小于它的非零特征值的个数
一个特征值可以有多个特征向量
在实数范围内,可能没有特征值和特征向量,但是在复数范围内,一定有,且有无穷多个特征向量
几何意义是:
方阵可以看作是对空间的一种线性变换,特征向量就是变化后方向保持不变的向量,特征值就是特征向量在变换后放缩的倍率。
行列式是平行四边形包围的面积,如果为负,就类似于平面翻转
Gram–Schmidt process
施密特正交法用于在欧氏空间中,给定线性无关向量组求标准正交基的过程
给定v,求e
$$ {\displaystyle \operatorname {proj} _{\mathbf {u} }(\mathbf {v} )={\frac {\langle \mathbf {u} ,\mathbf {v} \rangle }{\langle \mathbf {u} ,\mathbf {u} \rangle }}{\mathbf {u} }} $$
$$ {\displaystyle {\begin{aligned}\mathbf{u} _{1}&=\mathbf {v} _{1},&\mathbf {e} _{1}&={\frac {\mathbf {u} _{1}}{|\mathbf {u} _{1}|}}\\\mathbf {u} _{2}&=\mathbf {v} _{2}-\operatorname {proj} _{\mathbf {u} _{1}}(\mathbf {v} _{2}),&\mathbf {e} _{2}&={\frac {\mathbf {u} _{2}}{|\mathbf {u} _{2}|}}\\\mathbf {u} _{3}&=\mathbf {v} _{3}-\operatorname {proj} _{\mathbf {u} _{1}}(\mathbf {v} _{3})-\operatorname {proj} _{\mathbf {u} _{2}}(\mathbf {v} _{3}),&\mathbf {e} _{3}&={\frac {\mathbf {u} _{3}}{|\mathbf {u} _{3}|}}\\\mathbf {u} _{4}&=\mathbf {v} _{4}-\operatorname {proj} _{\mathbf {u} _{1}}(\mathbf {v} _{4})-\operatorname {proj} _{\mathbf {u} _{2}}(\mathbf {v} _{4})-\operatorname {proj} _{\mathbf {u} _{3}}(\mathbf {v} _{4}),&\mathbf {e} _{4}&={\mathbf {u} _{4} \over |\mathbf {u} _{4}|}\\&{}\ \ \vdots &&{}\ \ \vdots \\\mathbf {u} _{k}&=\mathbf {v} _{k}-\sum _{j=1}^{k-1}\operatorname {proj} _{\mathbf {u} _{j}}(\mathbf {v} _{k}),&\mathbf {e} _{k}&={\frac {\mathbf {u} _{k}}{|\mathbf {u} _{k}|}}\end{aligned}}} $$
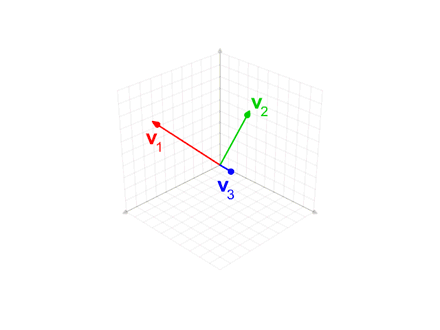
Gaussian elimination
Introduction
高斯消元可以用来求线性方程组的解,矩阵的秩,方阵的行列式,可逆矩阵的逆等等。
高斯消元使用初等行变换(elementary row operations),使矩阵有更多的0元素
初等行变换有3种
- 交换两行
- 对某一行乘上任意非零数
- 将某一行的倍数加到另一行(减同理)
高斯消元首先将矩阵变成行阶梯形式(row echelon form),此时可以判断方程无解、有唯一解、无穷多解,然后再变成最简行阶梯形式(reduced row echelon form),此时可以求出具体的解,转化到最简行阶梯矩阵的过程又叫Gauss–Jordan elimination
首项系数(leading coefficients)是每一行的第一个非0数,比如下面红色的数
$$ \left[\begin{array}{cccc} 0 & \color{red}{2} & 1 & -1\\0 & 0 & \color{red}3 & 1\\0 & 0 & 0 & 0 \end{array}\right] $$
最简行阶梯形式就是首项系数为1,首项系数所在的每一列,除了它以外全是0
高斯消元的过程如下
$$ \left[\begin{array}{cccc} 1 & 3 & 1 & 9 \\1 & 1 & -1 & 1 \\3 & 11 & 5 & 35 \end{array}\right] \rightarrow\left[\begin{array}{cccc} 1 & 3 & 1 & 9 \\0 & -2 & -2 & -8 \\0 & 2 & 2 & 8 \end{array}\right] \rightarrow\left[\begin{array}{cccc} 1 & 3 & 1 & 9 \\0 & -2 & -2 & -8 \\0 & 0 & 0 & 0 \end{array}\right] \rightarrow\left[\begin{array}{cccc} 1 & 0 & -2 & -3 \\0 & 1 & 1 & 4 \\0 & 0 & 0 & 0 \end{array}\right] $$
Example
假设要求解的方程组为
$$ {\displaystyle {\begin{alignedat}{4}2x&{}+{}&y&{}-{}&z&{}={}&8&\qquad (L_{1})\\-3x&{}-{}&y&{}+{}&2z&{}={}&-11&\qquad (L_{2})\\-2x&{}+{}&y&{}+{}&2z&{}={}&-3&\qquad (L_{3})\end{alignedat}}} $$
高斯消元的过程如下
System of equations | Row operations | Augmented matrix |
---|---|---|
$${\displaystyle {\begin{alignedat}{4}2x&{}+{}&y&{}-{}&z&{}={}&8&\\-3x&{}-{}&y&{}+{}&2z&{}={}&-11&\\-2x&{}+{}&y&{}+{}&2z&{}={}&-3&\end{alignedat}}}$$ | $${\displaystyle \left[{\begin{array}{rrr|r}2&1&-1&8\\-3&-1&2&-11\\-2&1&2&-3\end{array}}\right]}$$ | |
$${\displaystyle {\begin{alignedat}{4}2x&{}+{}&y&{}-{}&z&{}={}&8&\\&&{\tfrac {1}{2}}y&{}+{}&{\tfrac {1}{2}}z&{}={}&1&\\&&2y&{}+{}&z&{}={}&5&\end{alignedat}}}$$ | $${\displaystyle {\begin{aligned}L_{2}+{\tfrac {3}{2}}L_{1}&\to L_{2}\\L_{3}+L_{1}&\to L_{3}\end{aligned}}}$$ | $${\displaystyle \left[{\begin{array}{rrr|r}2&1&-1&8\\0&{\frac {1}{2}}&{\frac {1}{2}}&1\\0&2&1&5\end{array}}\right]}$$ |
$${\displaystyle {\begin{alignedat}{4}2x&{}+{}&y&{}-{}&z&{}={}&8&\\&&{\tfrac {1}{2}}y&{}+{}&{\tfrac {1}{2}}z&{}={}&1&\\&&&&-z&{}={}&1&\end{alignedat}}}$$ | $${\displaystyle L_{3}+-4L_{2}\to L_{3}}$$ | $${\displaystyle \left[{\begin{array}{rrr|r}2&1&-1&8\\0&{\frac {1}{2}}&{\frac {1}{2}}&1\\0&0&-1&1\end{array}}\right]}$$ |
$${\displaystyle {\begin{alignedat}{4}2x&{}+{}&y&&&{}={}7&\\&&{\tfrac {1}{2}}y&&&{}={}{\tfrac {3}{2}}&\\&&&{}-{}&z&{}={}1&\end{alignedat}}}$$ | $${\displaystyle {\begin{aligned}L_{2}+{\tfrac {1}{2}}L_{3}&\to L_{2}\\L_{1}-L_{3}&\to L_{1}\end{aligned}}}$$ | $${\displaystyle \left[{\begin{array}{rrr|r}2&1&0&7\\0&{\frac {1}{2}}&0&{\frac {3}{2}}\\0&0&-1&1\end{array}}\right]}$$ |
$${\displaystyle {\begin{alignedat}{4}2x&{}+{}&y&\quad &&{}={}&7&\\&&y&\quad &&{}={}&3&\\&&&\quad &z&{}={}&-1&\end{alignedat}}}$$ | $${\displaystyle {\begin{aligned}2L_{2}&\to L_{2}\\-L_{3}&\to L_{3}\end{aligned}}}$$ | $${\displaystyle \left[{\begin{array}{rrr|r}2&1&0&7\\0&1&0&3\\0&0&1&-1\end{array}}\right]}$$ |
$${\displaystyle {\begin{alignedat}{4}x&\quad &&\quad &&{}={}&2&\\&\quad &y&\quad &&{}={}&3&\\&\quad &&\quad &z&{}={}&-1&\end{alignedat}}}$$ | $${\displaystyle {\begin{aligned}L_{1}-L_{2}&\to L_{1}\\{\tfrac {1}{2}}L_{1}&\to L_{1}\end{aligned}}}$$ | $${\displaystyle \left[{\begin{array}{rrr|r}1&0&0&2\\0&1&0&3\\0&0&1&-1\end{array}}\right]}$$ |
Applications
Computing determinants
Finding the inverse of a matrix
Computing ranks and bases
LU decomposition
Introduction
LU分解是把方阵A分成L乘上U,L是下三角矩阵(lower triangular matrix),U是上三角矩阵(upper triangular matrix)
$$ {\displaystyle A=LU} $$
$$ {\displaystyle {\begin{bmatrix}a_{11}&a_{12}&a_{13}\\a_{21}&a_{22}&a_{23}\\a_{31}&a_{32}&a_{33}\end{bmatrix}}={\begin{bmatrix}\ell {11}&0&0\\\ell {21}&\ell {22}&0\\\ell {31}&\ell {32}&\ell {33}\end{bmatrix}}{\begin{bmatrix}u{11}&u{12}&u{13}\\0&u{22}&u{23}\\0&0&u{33}\end{bmatrix}}} $$
只有对A进行适当的排列才能分解成功,比如a11=l11*u11,如果a11=0,那么l11和u11必然有一个为0,这样L或者U就是奇异矩阵,那么A不可能非奇异。
有时候我们需要对A进行一定的重排列才可以LU分解
假设P是对A的行重排列,那么就可以这样分解,称为LUP分解
$$ {\displaystyle PA=LU} $$
假设Q是对A的列重排列,那么就可以这样分解
$$ {\displaystyle PAQ=LU} $$
还可以这样分解,D是对角矩阵,称为LDU分解
$$ {\displaystyle A=LDU} $$
Example
假设要分解这样的矩阵
$$ {\displaystyle {\begin{bmatrix}4&3\\6&3\end{bmatrix}}={\begin{bmatrix}\ell _{11}&0\\\ell _{21}&\ell _{22}\end{bmatrix}}{\begin{bmatrix}u_{11}&u_{12}\\0&u_{22}\end{bmatrix}}} $$
一种方法是写成线性方程组
$$ {\displaystyle {\begin{aligned}\ell _{11}\cdot u_{11}+0\cdot 0&=4\\\ell _{11}\cdot u_{12}+0\cdot u_{22}&=3\\\ell _{21}\cdot u_{11}+\ell _{22}\cdot 0&=6\\\ell _{21}\cdot u_{12}+\ell _{22}\cdot u_{22}&=3\end{aligned}}} $$
可以发现这个方程组有无穷多个解,有时候我们可以规定下三角矩阵的对角元为1,这样可以求出
$$ {\displaystyle {\begin{aligned}\ell _{11}=\ell _{22}&=1\\\ell _{21}&=1.5\\u_{11}&=4\\u_{12}&=3\\u_{22}&=-1.5\end{aligned}}} $$
$$ {\displaystyle {\begin{bmatrix}4&3\\6&3\end{bmatrix}}={\begin{bmatrix}1&0\\1.5&1\end{bmatrix}}{\begin{bmatrix}4&3\\0&-1.5\end{bmatrix}}} $$
Existence and uniqueness
Square matrices
Symmetric positive-definite matrices
General matrices
Rank factorization
将秩为r的m*n矩阵A,分解成CF,C是m*r的列满秩矩阵,F是r*n的行满秩矩阵
满秩分解可以求Moore-Penrose 伪逆(Moore-Penrose pseudoinverse),就是在方程组无解时,求最接近的解,在方程组无穷多解时求2范数最小的解
任何有限维矩阵都有满秩分解
如果${\textstyle A=C_{1}F_{1}}$是一个满秩分解,${\textstyle C_{2}=C_{1}R}$,${\textstyle F_{2}=R^{-1}F_{1}}$是另一个满秩分解,R是任意兼容维度下的可逆矩阵
Example
首先求A的最简行阶梯矩阵B,然后将B中每行非0首元(leading coefficient)所在的列在A中消去,产生矩阵C。将B中所有的全0行在B中消去产生矩阵F
考虑如下矩阵
$$ {\displaystyle A={\begin{bmatrix}1&3&1&4\\2&7&3&9\\1&5&3&1\\1&2&0&8\end{bmatrix}}\sim {\begin{bmatrix}1&0&-2&0\\0&1&1&0\\0&0&0&1\\0&0&0&0\end{bmatrix}}=B{\text{}}} $$
将A的第三列取出产生C,将B的最后一行去除产生F
$$ {\displaystyle C={\begin{bmatrix}1&3&4\\2&7&9\\1&5&1\\1&2&8\end{bmatrix}}{\text{,}}\qquad F={\begin{bmatrix}1&0&-2&0\\0&1&1&0\\0&0&0&1\end{bmatrix}}{\text{}}} $$
$$ {\displaystyle A={\begin{bmatrix}1&3&1&4\\2&7&3&9\\1&5&3&1\\1&2&0&8\end{bmatrix}}={\begin{bmatrix}1&3&4\\2&7&9\\1&5&1\\1&2&8\end{bmatrix}}{\begin{bmatrix}1&0&-2&0\\0&1&1&0\\0&0&0&1\end{bmatrix}}=CF{\text{}}} $$
Cholesky decomposition
一种常见的矩阵分解
适用于方阵,对称矩阵,正定矩阵,Hermite矩阵
Cholesky分解的效率大约是LU分解的两倍
对于Hermite正定矩阵A,它可以被分解成如下形式,其中L是下三角矩阵,且对角元为正实数,L*为L的共轭转置,所有的Hermite正定矩阵(当然包括实对称正定矩阵)都有唯一的Cholesky分解
$$ {\displaystyle \mathbf {A} =\mathbf {LL} ^{*}} $$
逆命题也成立,即如果一个矩阵能被分解成如上形式,它就是Hermite正定矩阵
特殊地,当A是实对称正定矩阵时,L是下三角矩阵,且对角元为正实数
$$ {\displaystyle \mathbf {A} =\mathbf {LL} ^{\mathsf {T}}} $$
LDL decomposition
由于Cholesky分解又叫平方根法,所以LDL分解又叫改进平方根法
分解形式如下,L是单位下三角矩阵(主对角线全为1),D是对角阵
这样分解的好处是避免提取平方根
$$ {\displaystyle \mathbf {A} =\mathbf {LDL} ^{*}} $$
如何从Cholesky分解转LDL分解?
假设正定矩阵A被分解成${\displaystyle \mathbf {A} =\mathbf {C} \mathbf {C} ^{*}}$,如果S是一个对角阵,包含了C的主对角元,那么${\displaystyle \mathbf {L} =\mathbf {C} \mathbf {S} ^{-1}}$,这样缩放了每一列元素,使对角线为1,${\displaystyle \mathbf {D} =\mathbf {S} ^{2}}$
如果A是正定矩阵,那么D的对角元都是正的
Example
对一个实对称矩阵做Cholesky分解
$$ \left(\begin{array}{rrr} 4 & 12 & -16 \\12 & 37 & -43 \\-16 & -43 & 98 \end{array}\right)=\left(\begin{array}{rrr} 2 & 0 & 0 \\6 & 1 & 0 \\-8 & 5 & 3 \end{array}\right)\left(\begin{array}{rrr} 2 & 6 & -8 \\0 & 1 & 5 \\0 & 0 & 3 \end{array}\right) $$
LDL分解
$$ \left(\begin{array}{rrr} 4 & 12 & -16 \\12 & 37 & -43 \\-16 & -43 & 98 \end{array}\right)=\left(\begin{array}{rrr} 1 & 0 & 0 \\3 & 1 & 0 \\-4 & 5 & 1 \end{array}\right)\left(\begin{array}{lll} 4 & 0 & 0 \\0 & 1 & 0 \\0 & 0 & 9 \end{array}\right)\left(\begin{array}{rrr} 1 & 3 & -4 \\0 & 1 & 5 \\0 & 0 & 1 \end{array}\right) $$
Computation
n维时间复杂度是O(n^3),Cholesky分解是一个迭代的过程,很适合计算机运算,此处只叙述LDL分解的迭代过程
当A是对称时
$$ {\displaystyle {\begin{aligned}\mathbf {A} =\mathbf {LDL} ^{\mathrm {T} }&={\begin{pmatrix}1&0&0\\L_{21}&1&0\\L_{31}&L_{32}&1\\\end{pmatrix}}{\begin{pmatrix}D_{1}&0&0\\0&D_{2}&0\\0&0&D_{3}\\\end{pmatrix}}{\begin{pmatrix}1&L_{21}&L_{31}\\0&1&L_{32}\\0&0&1\\\end{pmatrix}}\\[8pt]&={\begin{pmatrix}D_{1}&&(\mathrm {symmetric} )\\L_{21}D_{1}&L_{21}^{2}D_{1}+D_{2}&\\L_{31}D_{1}&L_{31}L_{21}D_{1}+L_{32}D_{2}&L_{31}^{2}D_{1}+L_{32}^{2}D_{2}+D_{3}.\end{pmatrix}}\end{aligned}}} $$
递归以下步骤
$$ {\displaystyle D_{j}=A_{jj}-\sum {k=1}^{j-1}L{jk}^{2}D_{k}} $$
$$ {\displaystyle L_{ij}={\frac {1}{D_{j}}}\left(A_{ij}-\sum {k=1}^{j-1}L{ik}L_{jk}D_{k}\right)\quad {\text{for }}i>j} $$
只要D的对角元能够一直保持非0,就能产生一个唯一的分解。如果A是实的,D和L也是实的
Applications
Linear least squares
Non-linear optimization
Monte Carlo simulation
Kalman filters
Matrix inversion
QR decomposition
适用于列线性无关的m*n的矩阵
分解形式,Q是m*m的酉矩阵,R是m*n的上三角矩阵
$$ {\displaystyle A=QR} $$
在很多时候,QR分解不唯一。但是如果A是满秩的,R是唯一的,且对角元都为正数,如果A是方阵,Q也是唯一的
QR分解可以很快地计算线性方程组${\displaystyle A\mathbf {x} =\mathbf {b} }$,因为Q是正交的,所以方程组可以化为${\displaystyle R\mathbf {x} =Q^{\mathsf {T}}\mathbf {b} }$,因为R是上三角矩阵,所以很好计算
接下来在实数空间上讨论
假设A是方阵
$$ {\displaystyle A=QR} $$
那么Q是正交矩阵,R是上三角矩阵,如果A是可逆的,且要求R对角元都为正数,则分解是唯一的
如果A是m*n的矩阵,m>=n,分解后Q是酉矩阵(实数下就是正交矩阵),R是上三角矩阵
Q是m*m,R是m*n,R1是n*n,0是(m-n)*n,Q1是m*n,Q2是m*(m-n),Q1和Q2都有正交列
$$ {\displaystyle A=QR=Q{\begin{bmatrix}R_{1}\\0\end{bmatrix}}={\begin{bmatrix}Q_{1}&Q_{2}\end{bmatrix}}{\begin{bmatrix}R_{1}\\0\end{bmatrix}}=Q_{1}R_{1}} $$
如果A是满秩(秩为n),且要求R1的对角元全为正数,则Q1和R1都是唯一的,但是一般Q2不是,R1是A*A(A是实的话就是A^TA)的Cholesky分解的上三角部分
Computing the QR decomposition
有很多种方法计算QR分解
Gram–Schmidt process
对于列满秩矩阵${\displaystyle A={\begin{bmatrix}\mathbf {a} _{1}&\cdots &\mathbf {a} _{n}\end{bmatrix}}}$,定义向量v和w的内积${\displaystyle \langle \mathbf {v} ,\mathbf {w} \rangle =\mathbf {v} ^{\textsf {T}}\mathbf {w} }$,定义投影${\displaystyle \operatorname {proj} _{\mathbf {u} }\mathbf {a} ={\frac {\left\langle \mathbf {u} ,\mathbf {a} \right\rangle }{\left\langle \mathbf {u} ,\mathbf {u} \right\rangle }}{\mathbf {u} }}$
施密特正交化过程为
$$ {\displaystyle {\begin{aligned}\mathbf {u} _{1}&=\mathbf {a} _{1},&\mathbf {e} _{1}&={\frac {\mathbf {u} _{1}}{\|\mathbf {u} _{1}\|}}\\\mathbf {u} _{2}&=\mathbf {a} _{2}-\operatorname {proj} _{\mathbf {u} _{1}}\mathbf {a} _{2},&\mathbf {e} _{2}&={\frac {\mathbf {u} _{2}}{\|\mathbf {u} _{2}\|}}\\\mathbf {u} _{3}&=\mathbf {a} _{3}-\operatorname {proj} _{\mathbf {u} _{1}}\mathbf {a} _{3}-\operatorname {proj} _{\mathbf {u} _{2}}\mathbf {a} _{3},&\mathbf {e} _{3}&={\frac {\mathbf {u} _{3}}{\|\mathbf {u} _{3}\|}}\\&\;\;\vdots &&\;\;\vdots \\\mathbf {u} _{k}&=\mathbf {a} _{k}-\sum _{j=1}^{k-1}\operatorname {proj} _{\mathbf {u} _{j}}\mathbf {a} _{k},&\mathbf {e} _{k}&={\frac {\mathbf {u} _{k}}{\|\mathbf {u} _{k}\|}}\end{aligned}}} $$
在此基础上,求A的各列向量
$$ {\displaystyle {\begin{aligned}\mathbf {a} _{1}&=\left\langle \mathbf {e} _{1},\mathbf {a} _{1}\right\rangle \mathbf {e} _{1}\\\mathbf {a} _{2}&=\left\langle \mathbf {e} _{1},\mathbf {a} _{2}\right\rangle \mathbf {e} _{1}+\left\langle \mathbf {e} _{2},\mathbf {a} _{2}\right\rangle \mathbf {e} _{2}\\\mathbf {a} _{3}&=\left\langle \mathbf {e} _{1},\mathbf {a} _{3}\right\rangle \mathbf {e} _{1}+\left\langle \mathbf {e} _{2},\mathbf {a} _{3}\right\rangle \mathbf {e} _{2}+\left\langle \mathbf {e} _{3},\mathbf {a} _{3}\right\rangle \mathbf {e} _{3}\\&\;\;\vdots \\\mathbf {a} _{k}&=\sum _{j=1}^{k}\left\langle \mathbf {e} _{j},\mathbf {a} _{k}\right\rangle \mathbf {e} _{j}\end{aligned}}} $$
其中${\displaystyle \left\langle \mathbf {e} _{i},\mathbf {a} _{i}\right\rangle =\left\|\mathbf {u} _{i}\right\|}$
那么Q和R可以写成如下形式
$$ {\displaystyle Q={\begin{bmatrix}\mathbf {e} _{1}&\cdots &\mathbf {e} _{n}\end{bmatrix}}} $$
$$ {\displaystyle R={\begin{bmatrix}\langle \mathbf {e} _{1},\mathbf {a} _{1}\rangle &\langle \mathbf {e} _{1},\mathbf {a} _{2}\rangle &\langle \mathbf {e} _{1},\mathbf {a} _{3}\rangle &\cdots &\langle \mathbf {e} _{1},\mathbf {a} _{n}\rangle \\0&\langle \mathbf {e} _{2},\mathbf {a} _{2}\rangle &\langle \mathbf {e} _{2},\mathbf {a} _{3}\rangle &\cdots &\langle \mathbf {e} _{2},\mathbf {a} _{n}\rangle \\0&0&\langle \mathbf {e} _{3},\mathbf {a} _{3}\rangle &\cdots &\langle \mathbf {e} _{3},\mathbf {a} _{n}\rangle \\\vdots &\vdots &\vdots &\ddots &\vdots \\0&0&0&\cdots &\langle \mathbf {e} _{n},\mathbf {a} _{n}\rangle \\\end{bmatrix}}} $$
Example
考虑如下矩阵
$$ {\displaystyle A={\begin{bmatrix}12&-51&4\\6&167&-68\\-4&24&-41\end{bmatrix}}} $$
用施密特正交法可以计算出
$$ {\displaystyle {\begin{aligned}U={\begin{bmatrix}\mathbf {u} _{1}&\mathbf {u} _{2}&\mathbf {u} _{3}\end{bmatrix}}&={\begin{bmatrix}12&-69&-58/5\\6&158&6/5\\-4&30&-33\end{bmatrix}}\\Q={\begin{bmatrix}{\frac {\mathbf {u} _{1}}{\|\mathbf {u} _{1}\|}}&{\frac {\mathbf {u} _{2}}{\|\mathbf {u} _{2}\|}}&{\frac {\mathbf {u} _{3}}{\|\mathbf {u} _{3}\|}}\end{bmatrix}}&={\begin{bmatrix}6/7&-69/175&-58/175\\3/7&158/175&6/175\\-2/7&6/35&-33/35\end{bmatrix}}\end{aligned}}} $$
$$ {\displaystyle {\begin{aligned}Q^{\textsf {T}}A&=Q^{\textsf {T}}Q\,R=R\\R&=Q^{\textsf {T}}A={\begin{bmatrix}14&21&-14\\0&175&-70\\0&0&35\end{bmatrix}}\end{aligned}}} $$
RQ分解就是把列看成行
Householder reflections
Connection to a determinant or a product of eigenvalues
$$ {\displaystyle \det A=\det Q\det R} $$
当Q的行列式为1时,rii是主对角元,也是特征值
$$ {\displaystyle \det A=\det R=\prod _{i}r_{ii}} $$
Singular value decomposition
适用于m*n的矩阵,分解形式
$$ {\displaystyle A=UDV^{*}} $$
D是非负的对角阵,U和V是酉矩阵(实数上就是正交矩阵)
D的对角元称为A的奇异值
A的奇异值是唯一的,U和V不一定唯一
在有些地方把奇异值分解的形式写成
$$ {\displaystyle \ \mathbf {M} =\mathbf {U\Sigma V^{*}} \ } $$
其中,矩阵的大小为
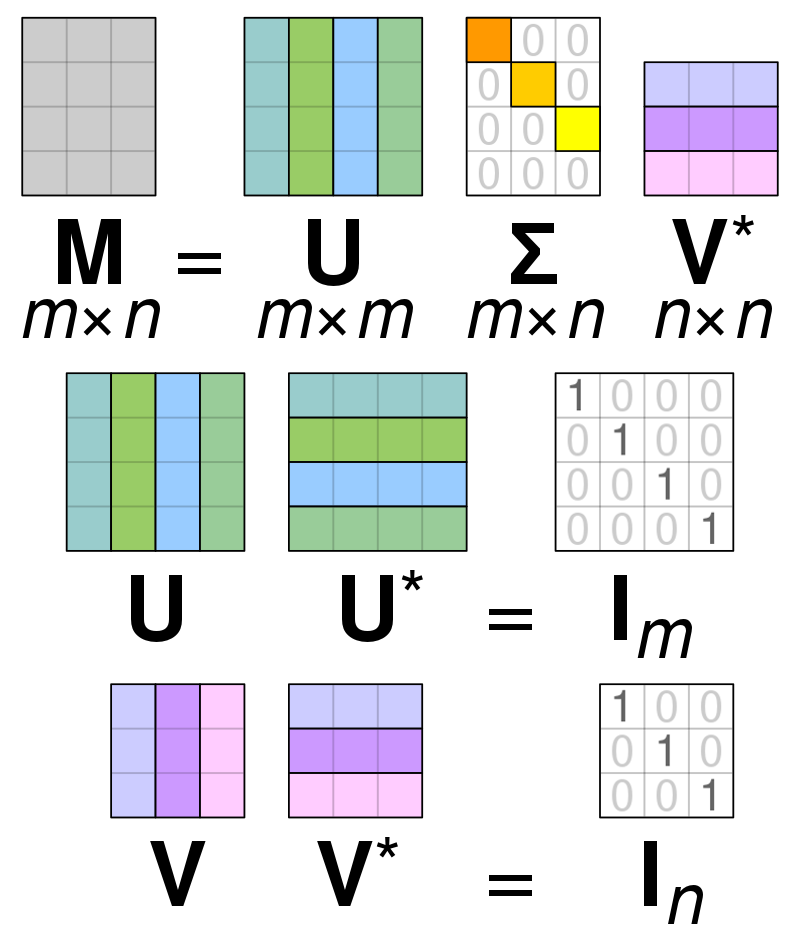
奇异值分解的几何意义是
对于M在空间上所做的变换,U将空间进行旋转,Σ将空间进行缩放,V*再将空间进行旋转,Σ的对角元就是缩放的比例
如果M的行列式是正的,那么U,V*可以都带反射(reflection)(就是将一张纸翻过来),或者都不带反射,如果M的行列式是负的,那么U或V*带反射
如果M不是方阵,假设是m*n的,几何意义可以看作是n维空间到m维空间的变换,U和V*分别作用于m维和n维空间,Σ除了对min(m,n)维空间进行缩放后,在其他维度要补0,以便后续的计算
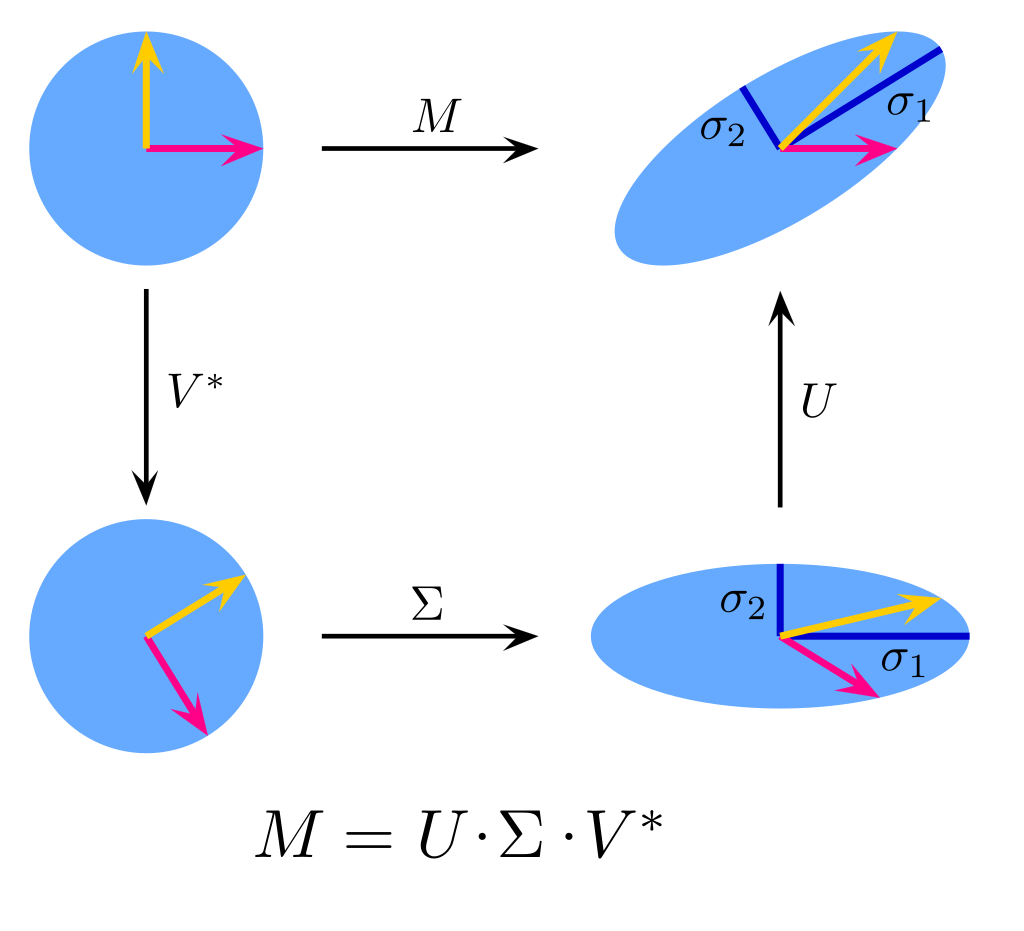
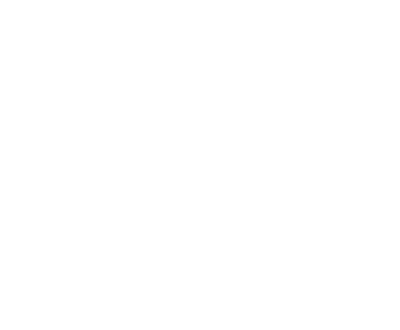
Example
$$ \mathbf {M} ={\begin{bmatrix}1&0&0&0&2\\0&0&3&0&0\\0&0&0&0&0\\0&2&0&0&0\end{bmatrix}} $$
$$ {\displaystyle {\begin{aligned}\mathbf {U} &={\begin{bmatrix}\color {Green}0&\color {Blue}-1&\color {Cyan}0&\color {Emerald}0\\\color {Green}-1&\color {Blue}0&\color {Cyan}0&\color {Emerald}0\\\color {Green}0&\color {Blue}0&\color {Cyan}0&\color {Emerald}-1\\\color {Green}0&\color {Blue}0&\color {Cyan}-1&\color {Emerald}0\end{bmatrix}}\\[6pt]{\boldsymbol {\Sigma }}&={\begin{bmatrix}3&0&0&0&\color {Gray}{\mathit {0}}\\0&{\sqrt {5}}&0&0&\color {Gray}{\mathit {0}}\\0&0&2&0&\color {Gray}{\mathit {0}}\\0&0&0&\color {Red}\mathbf {0} &\color {Gray}{\mathit {0}}\end{bmatrix}}\\[6pt]\mathbf {V} ^{*}&={\begin{bmatrix}\color {Violet}0&\color {Violet}0&\color {Violet}-1&\color {Violet}0&\color {Violet}0\\\color {Plum}-{\sqrt {0.2}}&\color {Plum}0&\color {Plum}0&\color {Plum}0&\color {Plum}-{\sqrt {0.8}}\\\color {Magenta}0&\color {Magenta}-1&\color {Magenta}0&\color {Magenta}0&\color {Magenta}0\\\color {Orchid}0&\color {Orchid}0&\color {Orchid}0&\color {Orchid}1&\color {Orchid}0\\\color {Purple}-{\sqrt {0.8}}&\color {Purple}0&\color {Purple}0&\color {Purple}0&\color {Purple}{\sqrt {0.2}}\end{bmatrix}}\end{aligned}}} $$
另一个V*
$$ {\displaystyle \mathbf {V} ^{*}={\begin{bmatrix}\color {Violet}0&\color {Violet}1&\color {Violet}0&\color {Violet}0&\color {Violet}0\\\color {Plum}0&\color {Plum}0&\color {Plum}1&\color {Plum}0&\color {Plum}0\\\color {Magenta}{\sqrt {0.2}}&\color {Magenta}0&\color {Magenta}0&\color {Magenta}0&\color {Magenta}{\sqrt {0.8}}\\\color {Orchid}{\sqrt {0.4}}&\color {Orchid}0&\color {Orchid}0&\color {Orchid}{\sqrt {0.5}}&\color {Orchid}-{\sqrt {0.1}}\\\color {Purple}-{\sqrt {0.4}}&\color {Purple}0&\color {Purple}0&\color {Purple}{\sqrt {0.5}}&\color {Purple}{\sqrt {0.1}}\end{bmatrix}}} $$
SVD分解的过程参考此处
Schur complement
舒尔补的作用是将p+q维矩阵的求逆变成p维和q维矩阵的求逆,降低运算时间
假设A、B、C、D矩阵为 p × p, p × q, q × p, q × q
$$ {\displaystyle M=\left[{\begin{matrix}A&B\\C&D\end{matrix}}\right]} $$
假设D是可逆的(即非奇异),则D在M中的舒尔补为
$$ {\displaystyle M/D:=A-BD^{-1}C} $$
假设A是可逆的,则A在M中的舒尔补为
$$ {\displaystyle M/A:=D-CA^{-1}B} $$
舒尔补在高斯消元中有用到,为了使M变成上三角矩阵,可以右乘一个下三角矩阵
$$ {\displaystyle {\begin{aligned}&M={\begin{bmatrix}A&B\\C&D\end{bmatrix}}\quad \to \quad {\begin{bmatrix}A&B\\C&D\end{bmatrix}}{\begin{bmatrix}I_{p}&0\\-D^{-1}C&I_{q}\end{bmatrix}}={\begin{bmatrix}A-BD^{-1}C&B\\0&D\end{bmatrix}}\end{aligned}}} $$
Ip是p阶单位阵,可以发现最后矩阵的左上角出现了D在M中的舒尔补
将这个结果左乘一个矩阵后转化为对角阵
$$ {\displaystyle {\begin{aligned}&{\begin{bmatrix}A-BD^{-1}C&B\\0&D\end{bmatrix}}\quad \to \quad {\begin{bmatrix}I_{p}&-BD^{-1}\\0&I_{q}\end{bmatrix}}{\begin{bmatrix}A-BD^{-1}C&B\\0&D\end{bmatrix}}={\begin{bmatrix}A-BD^{-1}C&0\\0&D\end{bmatrix}}\end{aligned}}} $$
由此可以引导出M的LDU分解
$$ {\displaystyle {\begin{aligned}M&={\begin{bmatrix}A&B\\C&D\end{bmatrix}}={\begin{bmatrix}I_{p}&BD^{-1}\\0&I_{q}\end{bmatrix}}{\begin{bmatrix}A-BD^{-1}C&0\\0&D\end{bmatrix}}{\begin{bmatrix}I_{p}&0\\D^{-1}C&I_{q}\end{bmatrix}}\end{aligned}}} $$
所以M的逆可以由D的逆和舒尔补的逆组成
$$ {\displaystyle {\begin{aligned}M^{-1}={\begin{bmatrix}A&B\\C&D\end{bmatrix}}^{-1}={}&\left({\begin{bmatrix}I_{p}&BD^{-1}\\0&I_{q}\end{bmatrix}}{\begin{bmatrix}A-BD^{-1}C&0\\0&D\end{bmatrix}}{\begin{bmatrix}I_{p}&0\\D^{-1}C&I_{q}\end{bmatrix}}\right)^{-1}\\={}&{\begin{bmatrix}I_{p}&0\\-D^{-1}C&I_{q}\end{bmatrix}}{\begin{bmatrix}\left(A-BD^{-1}C\right)^{-1}&0\\0&D^{-1}\end{bmatrix}}{\begin{bmatrix}I_{p}&-BD^{-1}\\0&I_{q}\end{bmatrix}}\\[4pt]={}&{\begin{bmatrix}\left(A-BD^{-1}C\right)^{-1}&-\left(A-BD^{-1}C\right)^{-1}BD^{-1}\\-D^{-1}C\left(A-BD^{-1}C\right)^{-1}&D^{-1}+D^{-1}C\left(A-BD^{-1}C\right)^{-1}BD^{-1}\end{bmatrix}}\\[4pt]={}&{\begin{bmatrix}\left(M/D\right)^{-1}&-\left(M/D\right)^{-1}BD^{-1}\\-D^{-1}C\left(M/D\right)^{-1}&D^{-1}+D^{-1}C\left(M/D\right)^{-1}BD^{-1}\end{bmatrix}}\end{aligned}}} $$
这是一种形式,可以交换A和D来推导出另一种形式
Properties
假设p和q都是1,AD-BC不为0
$$ M^{{-1}}={\frac {1}{AD-BC}}\left[{\begin{matrix}D&-B\\-C&A\end{matrix}}\right] $$
当A是可逆的,那么
$$ {\displaystyle {\begin{aligned}M&={\begin{bmatrix}A&B\\C&D\end{bmatrix}}={\begin{bmatrix}I_{p}&0\\CA^{-1}&I_{q}\end{bmatrix}}{\begin{bmatrix}A&0\\0&D-CA^{-1}B\end{bmatrix}}{\begin{bmatrix}I_{p}&A^{-1}B\\0&I_{q}\end{bmatrix}},\\[4pt]M^{-1}&={\begin{bmatrix}A^{-1}+A^{-1}B(M/A)^{-1}CA^{-1}&-A^{-1}B(M/A)^{-1}\\-(M/A)^{-1}CA^{-1}&(M/A)^{-1}\end{bmatrix}}\end{aligned}}} $$
当A(D)是可逆的,则
$$ {\displaystyle \det(M)=\det(A)\det \left(D-CA^{-1}B\right)}\\{\displaystyle \det(M)=\det(D)\det \left(A-BD^{-1}C\right)} $$
当D是可逆的,则
$$ {\displaystyle \operatorname {rank} (M)=\operatorname {rank} (D)+\operatorname {rank} \left(A-BD^{-1}C\right)} $$
Application to solving linear equations
假设要解这样的线性方程
$$ {\displaystyle {\begin{bmatrix}A&B\\C&D\end{bmatrix}}{\begin{bmatrix}x\\y\end{bmatrix}}={\begin{bmatrix}u\\v\end{bmatrix}}} $$
假设A是可逆的,可以得到
$$ {\displaystyle x=A^{-1}(u-By)} $$
将上式代入可以得到
$$ {\displaystyle \left(D-CA^{-1}B\right)y=v-CA^{-1}u} $$
定义A的舒尔补
$$ {\displaystyle S\ {\overset {\underset {\mathrm {def} }{}}{=}}\ D-CA^{-1}B} $$
x和y可以变成
$$ {\displaystyle y=S^{-1}\left(v-CA^{-1}u\right)} $$
$$ {\displaystyle x=\left(A^{-1}+A^{-1}BS^{-1}CA^{-1}\right)u-A^{-1}BS^{-1}v} $$
可以写成如下形式
$$ {\displaystyle {\begin{bmatrix}x\\y\end{bmatrix}}={\begin{bmatrix}A^{-1}+A^{-1}BS^{-1}CA^{-1}&-A^{-1}BS^{-1}\\-S^{-1}CA^{-1}&S^{-1}\end{bmatrix}}{\begin{bmatrix}u\\v\end{bmatrix}}} $$
大矩阵的逆可以分解成小矩阵的逆
$$ {\displaystyle {\begin{bmatrix}A&B\\C&D\end{bmatrix}}^{-1}={\begin{bmatrix}A^{-1}+A^{-1}BS^{-1}CA^{-1}&-A^{-1}BS^{-1}\\-S^{-1}CA^{-1}&S^{-1}\end{bmatrix}}={\begin{bmatrix}I_{p}&-A^{-1}B\\&I_{q}\end{bmatrix}}{\begin{bmatrix}A^{-1}&\\&S^{-1}\end{bmatrix}}{\begin{bmatrix}I_{p}&\\-CA^{-1}&I_{q}\end{bmatrix}}} $$
Something confusing
- 矩阵左乘一个行向量的几何意义
Reference
无水印的图来自wikipedia